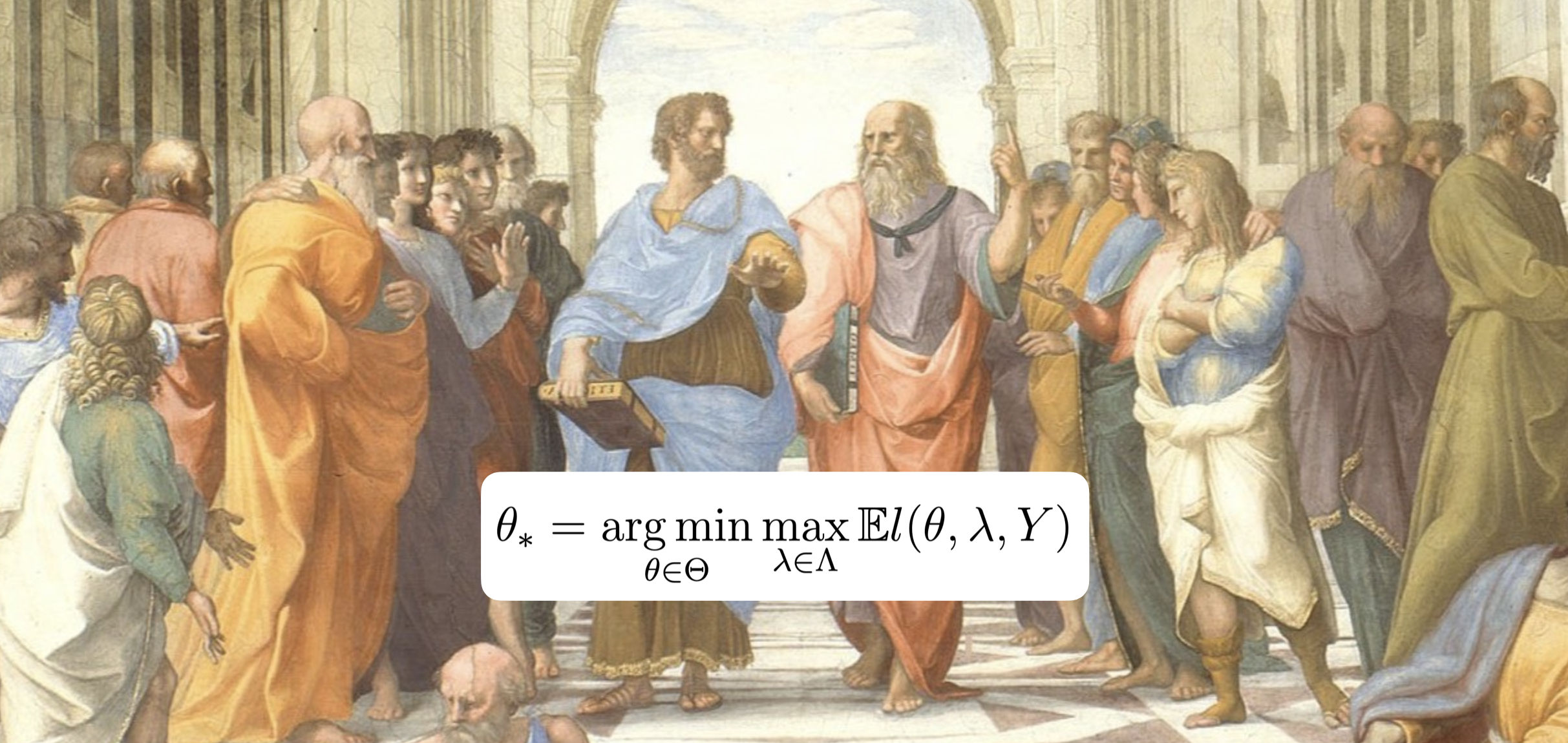
Adversarial Estimators
This paper develops the statistical theory (i.e. derives convergence rates and asymptotic normality) of adversarial estimators, which estimate quantities of interest by pitting two models against each other. The paper proves traditional statistical guarantees even for semiparametric settings involving deep neural networks, and points out interesting information-theoretic connections between various estimators: from traditional econometric methods such as Empirical Likelihood, over Generative AI methods such as GANs, to modern nonparametric causal inference estimators.